In our daily life, we encounter various people, objects, or environmental context that can evoke memories of unpleasant events. Some of us might be able to quickly move on from these memories, whereas others might get stuck repeatedly thinking about them. The tendency to repeatedly think about stressors is called rumination, which is closely linked to our experience of distress and maintains many clinical disorders, such as depression and anxiety. Mindfulness-based interventions are effective in reducing rumination. However, we do not know what specific aspects of ruminative thoughts are reduced by these interventions, due to constraints in common self-report measures. I propose to develop a new paradigm to capture fine-grained information about what people are ruminating about in the moment that it happens, how long they spend ruminating, and how often they switch between rumination and other thoughts. I will use machine learning techniques to analyze these patterns of rumination and advance our knowledge in how mindfulness training affects these patterns. This knowledge will address issues of disconnection at the individual and interpersonal levels, as it will guide us to design interventions to help people disengage from unhelpful repetitive thinking and reconnect with the present moment and people around them.
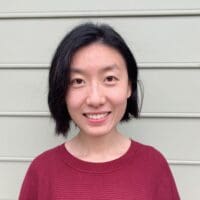